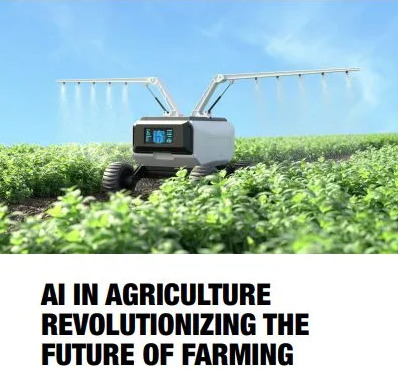
Introducing AI in Agriculture
Agriculture has always been the foundation of human civilization, providing sustenance for our ever-growing population. However, traditional farming methods have often struggled with inefficiencies, resource wastage, and sustainability issues. The integration of Artificial Intelligence (AI) into agriculture has emerged as a trans-formative solution to address these challenges.
AI leverages advanced technologies such as machine learning, computer vision, and data analytic to enhance farming practices. Its problem-solving capabilities and data-driven insights offer new avenues for modern agriculture.
The Evolution of Agriculture
Agriculture has come a long way since the days of manual labor and basic tools. The shift towards mechanization marked a significant milestone, improving productivity and reducing the strain on labor. AI is the next evolutionary leap in this journey, ushering in the era of smart farming.
The Role of Artificial Intelligence
Artificial Intelligence encompasses a spectrum of technologies that work together to optimize agriculture. Machine learning algorithms analyze vast datasets to make predictions and offer insights. Computer vision enables machines to recognize and respond to visual data, while data analytic assists in decision-making.
Precision Agriculture
Precision agriculture is one of the most compelling applications of AI in the field. It involves the use of AI-powered machinery and sensors to precisely manage resources such as fertilizers, pesticides, and irrigation. By tailoring these inputs to the specific needs of each area of a field, farmers can maximize crop yields while minimizing resource waste. This not only improves efficiency but also contributes to sustainable agriculture.
AI-Powered Crop Monitoring
AI brings a new level of precision to crop monitoring. Drones equipped with AI systems can fly over fields, capturing high-resolution images and data. These images are then analyzed to identify areas of concern, such as signs of disease, pests, or nutrient deficiencies. With this early detection, farmers can take swift action to address issues, preventing crop loss and reducing the need for chemical treatments.
Enhanced Pest and Disease Management
AI plays a pivotal role in early pest and disease detection. By collecting data on factors like temperature, humidity, and plant health, AI algorithms can predict potential outbreaks. This proactive approach allows farmers to respond swiftly, often with targeted and environmentally friendly interventions. By reducing the reliance on chemical pesticides, AI contributes to sustainable farming practices.
Automation in Farming
The integration of AI-powered automation has streamlined many farming tasks. Autonomous vehicles and robots can perform repetitive and physically demanding activities with remarkable precision. Whether it’s planting, harvesting, or weeding, these machines save time and labor, making agriculture more efficient.
Improved Water Management
Effective water management is crucial for agriculture, especially in regions facing water scarcity. AI algorithms process data from weather forecasts, soil sensors, and crop conditions to optimize irrigation. By providing just the right amount of water when and where it’s needed, AI conserves this precious resource and reduces waste.
AI in Livestock Farming
AI isn’t limited to crops; it also benefits livestock farming. Facial recognition and health monitoring systems help farmers keep track of their animals’ well-being. Early detection of health issues and efficient feeding practices contribute to higher livestock productivity.
Sustainable Agriculture Practices
AI actively promotes sustainable agricultural practices. By reducing resource wastage, optimizing input usage, and minimizing environmental impact, it aligns with the principles of responsible farming. This not only benefits the environment but also ensures the long-term viability of the industry.
Challenges and Concerns
While the promise of AI in agriculture is vast, it comes with its share of challenges. The initial cost of adopting AI technology can be a barrier for some farmers. Additionally, there is a need for education and training to ensure that farmers can effectively use AI tools. Data security is also a concern, as sensitive agricultural data must be protected from cyber threats.
- Cost of Implementation: One of the foremost challenges of implementing AI in agriculture is the initial cost. The adoption of AI technologies often requires significant investments in hardware, software, and specialized equipment. Small-scale farmers may find it especially challenging to acquire and maintain these systems. The question of how to make AI more accessible and cost-effective for all farmers remains a significant concern.
- Education and Training: AI systems are powerful, but they require users to understand how to harness their potential. Many farmers, especially those in remote or under-served regions, may lack the necessary knowledge and training to effectively operate AI-driven equipment and interpret the data they produce. Bridging this education gap is crucial to ensure that AI is utilized to its fullest potential.
- Data Privacy and Security: Agriculture generates vast amounts of data, including sensitive information about crops, livestock, and farm operations. Protecting this data from cyber threats and ensuring privacy is a paramount concern. As AI relies on data for decision-making, any breach of security could have severe consequences, including financial losses and compromised competitive advantages.
- Compatibility and Integration: The agriculture sector is diverse, with a wide range of machinery and systems already in use. Integrating AI technologies with existing infrastructure can be a complex process. Ensuring that AI systems are compatible with older equipment and farm management software is a technical challenge that must be addressed.
More:
- Dependence on Connectivity: AI systems often require a reliable internet connection to function optimally. In rural areas with poor or no connectivity, this poses a significant challenge. Farmers in remote regions may find it difficult to access the real-time data and support that AI offers, limiting the benefits of these technologies.
- Ethical Concerns: As AI becomes more integrated into agriculture, ethical concerns emerge. For instance, the use of AI for animal welfare and the potential consequences of advanced gene editing technologies raise questions about the ethical boundaries of AI in agriculture. Balancing technological advancement with ethical considerations is a complex issue.
- Regulatory Hurdles: The regulatory environment for AI in agriculture is still evolving. Governments and regulatory bodies must create guidelines and standards to ensure the responsible and safe use of AI. Striking the right balance between promoting innovation and safeguarding consumer interests is a challenge that policymakers must navigate.
- Data Quality and Reliability: AI systems depend on high-quality and reliable data to make accurate predictions and recommendations. Inconsistent or inaccurate data can lead to flawed decision-making. Farmers need robust mechanisms to collect and maintain data quality, which can be demanding, particularly for small-scale operations.
- Overcoming Resistance to Change: Traditionally, farming has been deeply rooted in tradition. Convincing farmers to embrace AI and change long-established practices can be met with resistance. Demonstrating the clear benefits and the potential for increased productivity is crucial in overcoming this challenge.
- Environmental Concerns: While AI can contribute to sustainability in agriculture, there are environmental concerns related to its energy consumption and the use of AI-driven machinery. Finding environmentally friendly solutions and minimizing the carbon footprint of AI systems is a challenge that aligns with the broader goal of sustainable agriculture.
Live Illustration of AI in agriculture
The implementation of AI in agriculture has gained significant momentum in recent years, and there are several live examples that showcase its practical applications. Here is a list of live examples where AI is being used in agriculture:
- Blue River Technology’s See & Spray: Blue River Technology, a subsidiary of John Deere, has developed an AI-driven system that uses computer vision to identify and precisely target individual weeds. This technology reduces the need for herbicides, resulting in more efficient and sustainable farming.
- Agrobot’s Strawberry-Picking Robot: Agrobot’s AI-powered robot is designed to pick ripe strawberries selectively. It uses machine learning algorithms to identify ripe fruit and pick them gently, reducing both labor costs and fruit waste.
- Connecterra’s Ida, the AI-Powered Cow Collar: Ida is an AI-powered collar for cows that monitors their behavior and health. It uses machine learning to detect patterns in a cow’s behavior, helping farmers identify when an individual cow is in heat, sick, or not eating properly.
- FarmWise’s Weeding Robot: FarmWise has developed a weeding robot that uses computer vision and machine learning to identify and remove weeds from crop fields. The robot can reduce the need for chemical herbicides and manual labor.
- IBM’s Watson Decision Platform for Agriculture: IBM’s Watson Decision Platform for Agriculture offers AI-driven solutions for farmers. It provides real-time weather data, soil information, and predictive analytic to help farmers make informed decisions about planting, harvesting, and resource management.
- Gamaya’s Crop Monitoring: Gamaya offers a comprehensive crop monitoring solution that uses hyper spectral imaging and AI to provide detailed insights into crop health, disease detection, and yield prediction.
More:
- AeroFarms’ Vertical Farming: AeroFarms uses AI to optimize the growth conditions for crops in vertical farming. AI-controlled lighting, temperature, and humidity adjustments help produce high-quality crops efficiently in indoor environments.
- Taranis’ Precision Agriculture Platform: Taranis offers a precision agriculture platform that uses AI to analyze satellite and drone imagery. It helps farmers identify early signs of crop disease, pest infestations, and nutrient deficiencies, enabling timely intervention.
- Sentera’s FieldAgent: Sentera’s FieldAgent is an AI-driven software that provides detailed aerial imagery and analytics for precision agriculture. It helps farmers monitor crop health and make data-driven decisions to maximize yield.
- Bosch’s IoT-based Solutions: Bosch offers a range of AI-driven solutions for agriculture, including smart irrigation systems, environmental monitoring, and livestock tracking. These systems use sensors and AI to improve resource efficiency and animal welfare.
These live examples illustrate the diverse applications of AI in agriculture, from precision farming and pest control to livestock monitoring and sustainable practices. They represent a promising shift towards more efficient, sustainable, and data-driven farming practices.
The Future of AI in Agriculture
The future of AI in agriculture is incredibly promising. As technology continues to advance and AI becomes more accessible, its role in farming will only expand. With even more sophisticated AI systems, the agricultural sector is poised for greater efficiency, productivity, and sustainability.
In conclusion, AI in agriculture is a revolution that promises to transform the way we produce food. It’s not merely a technological advancement but a necessity for addressing the challenges of food production, resource management, and environmental sustainability. As AI continues to evolve, it will empower farmers to feed the world’s growing population while respecting the planet’s limits.