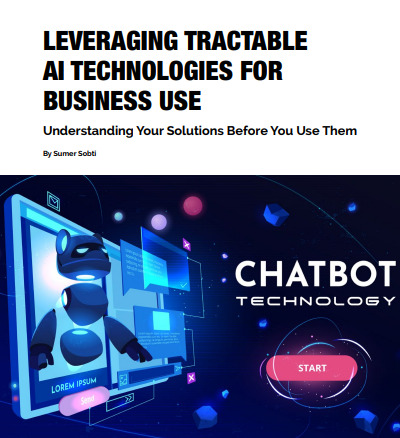
Understanding Your Solutions Before You Use Them
By Sumer Sobti
In 1698, Thomas Savery invented a suction pump to extract water from coal mines, using steam condensation. In 1712, Thomas Newcomen developed the piston, a precursor to the steam engine built by James Watt in 1764. These developments are considered the first industrial revolution, and signalled the advent of an era when machines began replacing human and animal effort. The setting up of factories and mass production assembly lines are said to be the second industrial revolution. Computers made their debut in the mid-20th century. This was the third industrial revolution, also a time when robotic manufacturing took its first tentative steps. Computers began to aid in design and manufacture, and analysed large volumes of data. As computer hardware and chips became more powerful, software marched in step, becoming increasingly sophisticated. Then a sweeping revolution in communication technology transformed information technology to a whole new level.
Industry 4.0
We are now in the middle of the fourth industrial revolution, the information age, with almost unlimited access to astounding computational ability and always-on high speed data and voice communication. Artificial Intelligence is the heart of this revolution. The next big thing in this era of course, is ChatGPT, OpenAI’s impressive Large Language Model (LLM) chatbot that uses natural language processing to answer questions and composes content on demand.
LLMs are a disruptive deep learning technology. They combine huge volumes of information accessed from various sources, with clever machine learning techniques, and generate a degree of knowledge supremacy that was the stuff of science fiction. Deep learning models use neural networks and self-supervised learning paradigms. They take input text and then predict the next token (smallest text unit), eventually generating entire text sequences based on the previous tokens. As ‘learning’ models, they evolve with every instance of ‘training’ or logic assertion provided to them.
The Problem with LLMs
Despite the impressive capability of deep neural network models, there are some problems. For one, deep learning models do not provide the basis for their outcomes. In neural networks, logic is a black box and the algorithm is blind-trained using large volumes of data. It is this repetitive training that sets the internal weights and parameters which function as the algorithm. No actual logic constructs are ever provided. Thus when a solution is generated, its logical basis remains unclear. LLMs also often give incorrect responses as they are built to accept training data without validation and lack the means to evaluate input data. This means that inherent errors and biases, or incompleteness of information is reflected in the output.
There are other problems too. LLMs can find answers to almost any query under the sun, as they scan and scrape vast amounts of data from third party sources across the globe. These third parties do not automatically authorize the commercial use of their data. This technology approach therefore raises ethical concerns. But if LLMs are expected to seek consent before using publically available data, the paradigm will become quite unsustainable.
Deep neural networks must be continuously re-trained to ensure their embedded knowledge is fully current. This is an extremely data-hungry prospect. Assured access to huge volumes of data is now becoming increasingly difficult. Governments across the world are hardening their stance over perceived IPR violations and are unwilling to allow the untrammeled use of third party data with putative infringement of copyright laws. The consequence is the emergence of data protection regimes like the GDPR and India’s own Digital Personal Data Protection Act, 2023. Till there is greater clarity, the legality of deep learning tools lies in a grey zone.
What AI can do for Businesses
Businesses must look for AI technologies that provide force multiplier effect, yet transparently explain how decisions are arrived at. Further, AI-based solutions work best only when they are custom-built for a specific business requirement. AI solutions created for one requirement simply cannot be used to solve another problem. Any attempt to do so will provide highly sub-optimal outcomes, if at all it works.
An AI paradigm that provides decision-making solutions using multiple input parameters, is Multi-Criteria Decision Making (MCDM). This AI model records the various courses of action or alternatives available to solve a problem, as defined by the user. It then carries out comparative evaluation between alternatives to arrive at the best one. To enable comparisons, each alternative has measurable attributes, and the comparison itself is done on the basis of user-defined criteria.
MCDM is ideal for Risk Assessment
MCDM is ideal for Risk Assessment, a field that evaluates the potential risks of a business process or planned activity, so that the least risky option may be adopted. This requires domain expertise to model the risks associated with each course of action or possible method of executing a business process. There is also a need to identify the attributes the business process seeks to maximize. Risk assessment must be done for each course of action, under the current set of circumstances. MCDM logic engines are vastly superior to humans in solving such complex problems, once the user defines the attributes and the criteria accurately.
Take a car production unit near Bangalore that wants to send its finished cars to retailers across North India. The cars can be sent by road in car-trailers (alternative 1), by train (alternative 2), or driven down (alternative 3). For such a problem, the MCDM reasoner is provided a set of weighted attributes for each option, e.g., delivery time, delivery cost per car, maximum number of cars transportable per day for each medium, etc.
The criteria for assessment would include the need to minimise: cost of transit, cost of delay, probability of material loss through accident, through theft, etc. The MCDM solution provides the best option while also providing insights from every process instance (delivery). These can be recorded as cases, which can be further used to create Case-Based Learning solutions that facilitate intelligent storage of the lessons learnt, to create institutionalized memory, which can be referred to later by businesses.
Use of Multi-Agent Systems (MAS)
The use of Multi-Agent Systems (MAS) for planning and resource management, is another AI-based technology that is a viable business investment. MAS involves the creation of multiple decision-making agents. Each agent is assigned a goal, which it must try and fulfil within its ecosystem. Agents interact and ‘negotiate’ with each other to arrive at a consensus amongst themselves.
Why are multi-agent systems useful? In any business environment, there is an undeniable need to fulfill contradictory requirements to meet business goals. MAS handles the complexity and provides an unbiased approach to solve the planning problem. Once agent goals have been defined, negotiation ranges provided and goal primacies set (so that agents prioritize higher for more important goals), the AI-engine handles the complexity of decision-making. Every agent interactively negotiates with other agents using inter se priorities, till a kind of homeostasis or equilibrium is reached, which at least partially satisfies all the agents.
How MAS works?
To illustrate how MAS works, we take the simplistic example of space optimization for urban planning, while planning a residential complex. An MAS agent is assigned for each of the undermentioned requirements, with goals assigned to them as under:
- Goal – Agent 1: Build flats only of size 2000 square feet (error margin 2%).
- Goal – Agent 2: Build only four flats per floor, as all residents want corner flats.
- Goal – Agent 3: Ensure ratio of built-up area to total area does not exceed 30%.
- Goal – Agent 4: Build a Clubhouse of at least 15,000 square feet.
- Goal – Agent 5: Build at least 400 flats for adequate return on investment.
- Goal – Agent 6: Ensure Floor Space Index of 145% as per building laws (inviolable).
Clearly, these are all conflicting requirements, and can be settled only through some compromise in each individual goal, so as to achieve the larger optima.
In complex planning problems, human endeavor often falls short when compared to well-designed AI solutions. For businesses that plan the movement of goods through multiple physical spaces that are available only for limited time windows, AI-based Path Planning and Scheduling algorithms provide the most optimal solutions.
Path Planning and Scheduling algorithms
Often complex business plans need to be changed to handle unforeseen failure or the sudden non-availability of planned-for resources. If the plan is already in motion, it can be a nightmare. AI–based Plan Repair algorithms help make changes to complex plans while minimizing the disruptive effect and limiting the adverse impact of the changes to the least number of entities within the plan ecosystem. Incremental Planning algorithms allow additions and extensions to existing plans, while minimising any potential adverse effects caused by the changes.
AI technologies offer exponentially greater efficiency to solve complex business problems. But AI is resource-hungry. To leverage it, organisations must be prepared to invest in expert human capital. Business leaders must also ensure that the technologies they use to solve their problems transparently explain themselves. So that the ultimate control vests with business leaders, not with the technologies they use.
About Author
Sumer Sobti works as a technology and business consultant. He has worked with the Centre for Artificial Intelligence & Robotics, Bangalore. His work on Net-Centric systems and Intelligent Systems design led to the definition of domain protocol frameworks for planning and decision supportproblems. He is also a qualified Software Test Manager, certified by the Standardisation Testing and Quality Certification Directorate, Ministry of Electronics and Information, Govt. of India. He currently lives and works in Bangalore.